How To Deal With Noise In Maritime Data?
In the maritime industry, the collection of vast amounts of data is both an opportunity and a challenge. While data can provide valuable insights into operations, not all data collected is accurate, leading to what is known as “noise.” This noise, or irrelevant and unwanted data, can distort analysis and decision-making. Here’s how to effectively manage and filter out noise in maritime data to ensure accuracy and reliability.
Understanding Noise in Maritime Data
Noise in data refers to information that is either irrelevant or unwanted. Irrelevant data may include information that does not pertain to the current context, such as details about sailing ships when focusing on cargo movements. Unwanted data, on the other hand, can include erroneous sensor readings or spoofed data from open sources. Both types of noise can interfere with accurate data analysis and decision-making.
Identifying and Filtering Noise
Define Relevance
Start by clearly defining what constitutes relevant data for your specific context. For instance, focus on cargo movements rather than unrelated maritime activities like sailing vessels.Detecting Erroneous Sensor Data
Sensors can occasionally produce incorrect readings. Implementing algorithms to detect and exclude these anomalies is crucial. Regular calibration and maintenance of sensors also help in minimizing erroneous data.Addressing Spoofed Data
When using open data, there is a risk of encountering spoofed or manipulated information. Employing robust validation mechanisms can help in identifying and filtering out such data before it contaminates your system.
These faulty information is quite often at the beginning. So, when collecting data from different sources, you want to make sure that unwanted data is being filtered out right away.
Detecting this kind of unwanted data and filtering it out is a part of the solution that we provide.
Richard van Klaveren
CTO & co-founder of Teqplay
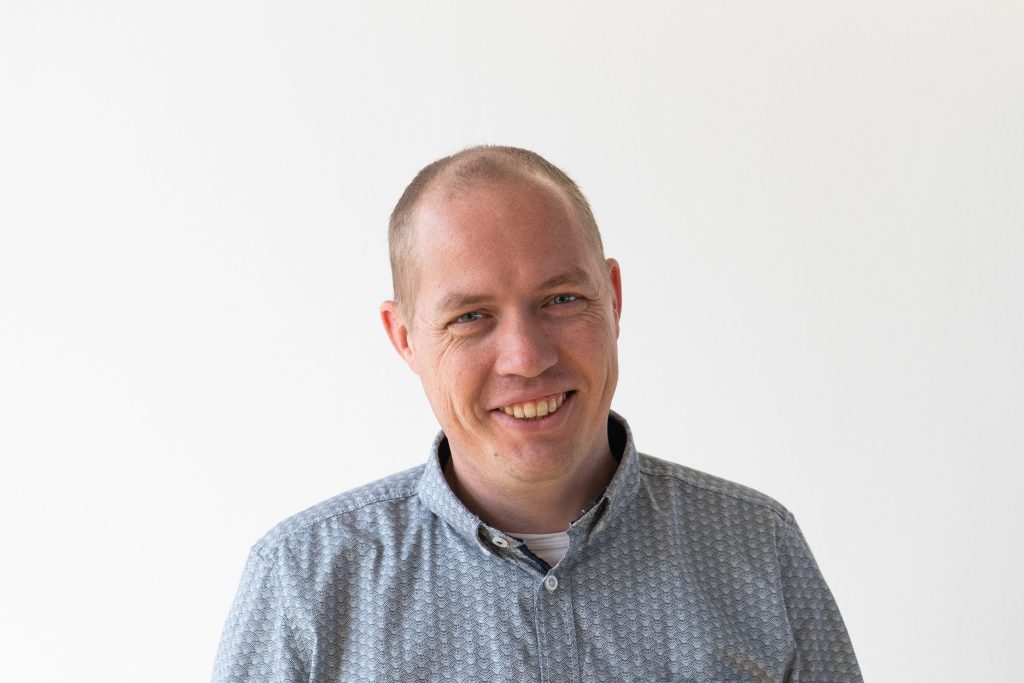
Managing Outliers
Outliers are data points that deviate significantly from other observations and can skew analysis results. For example, if you are analyzing port turnaround times, an outlier could be a vessel that docked unusually long due to unexpected circumstances. Identifying and filtering out such outliers ensures that your analysis remains accurate and relevant.
Quality Control Throughout the Data Pipeline
Ensuring data quality involves continuous monitoring and filtering throughout the data pipeline. From initial data collection to final analysis, maintaining rigorous quality control measures helps in keeping the data clean and reliable.
Leveraging Technology for Data Filtering
Advanced technologies such as machine learning and artificial intelligence can automate the process of detecting and filtering noise. These technologies can learn from historical data patterns to identify anomalies and irrelevant information, thereby improving the accuracy of your data insights.
Hear more from Teqplay’s maritime experts and industry thought leaders in our video podcast here.